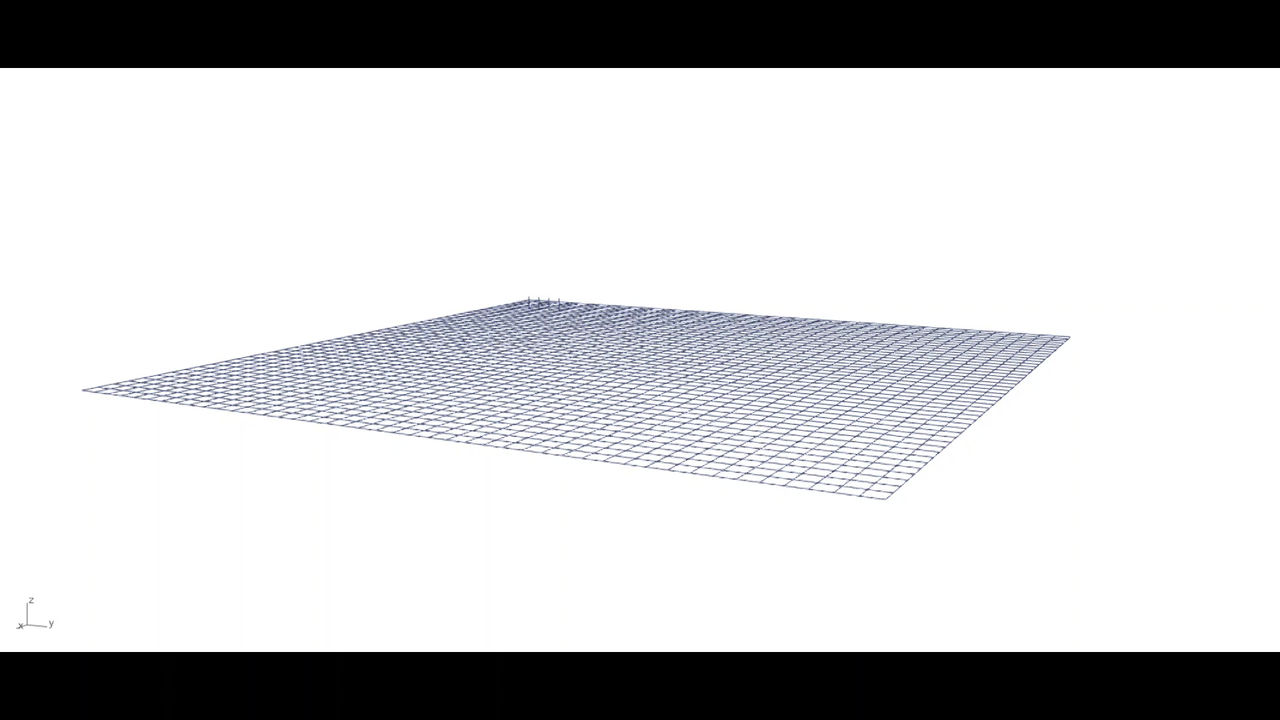
Predictive Analytics
STATS
*briefly
and influences
Neighbors
Defining the neighboring regions is important for both validating the data collected as well as the insights deduced from it. There are different kinds of neighboring conditions.
Clockwise from top-left: 1. Municipal neighborhoods 2. Yafo v.s TLV - coast v.s mainland 3. potentially flooded areas against population density 4. Grouping by "Polarity" value (how extreme ara local positive qualities and negative qualities (e.g all inhabitants have higher education AND there's a very high crime rate) 5. Walking distance from the site (using streets) 6. Grouping by architectural styles (e.g northern old modernist TlV, Southern Jaffa old modernist apt blocks).
Dependent variable
Our
The generalization of geometric objects, within the context of topological features, divides them according to their respective dimensions, i.e ‘simplices’. These are point (0-simplex), line (1-simplex), polygon (2-simplex) ,tetrahedron (3-simplex), and beyond.
When seeking to transform an agglomeration process, the smaller the size of the altered particle, the more diverse the agglomerative end will be. The urban fabric, a part-whole condition of such process, is comprised of different sized particles: blocks, streets, plots, buildings... Following "The course of dimensionality" we can think of a "building's"/:"mass" as the particle and attribute under the highest resolution and simplex degree while remaining comprehensible.
Realm of models is based on abstract universals
SIMPLE
PHENOMENON
Properties of the system for modeling
Possible relations (types and numbers)
COMPLEX PHENOMENON
Let's assume that we measure the complexity of a system (i.e real phenomenon) as a function of the number of its potential properties and the relations between those properties.
In this scenario, in comparison to a building, a chair is less complex. The same relation holds for a building in comparison to a city. As a result, by increasing the number of potential properties and their interrelationships, and consequently the exponential growth in the number of combinations, the space of modeling potential expands in an exponential manner.
As the number of dimensions grows, the amount of data that we need to generalize accurately grows exponentially.
Conclusions
Highest amounts of built masses are along the coast of TLV and mainland
-
44.72/55.28 %
-
47.46/52.54 %
-
42.46/57.54 %
-
32.74/67.26 %
-
40.76/59.24 %

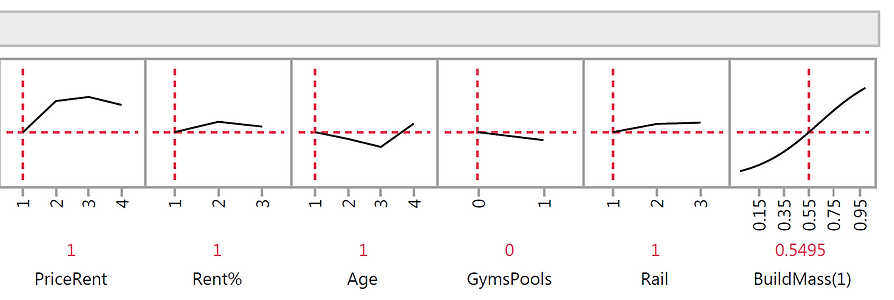